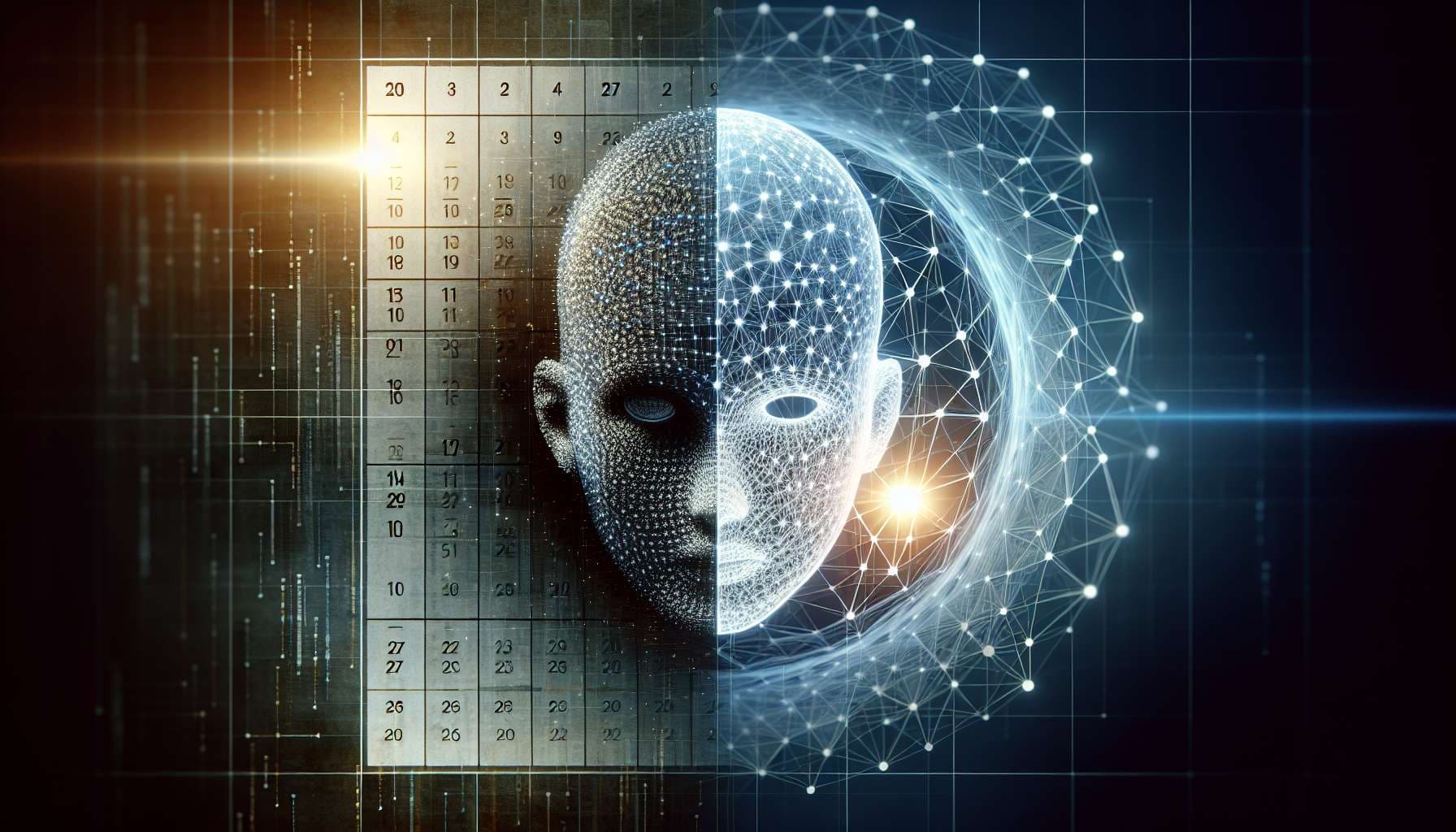
Meta's Coconut paper describes a new way to train AI models so that they reason in latent space. Coconut doesn't have to explicitly write its thoughts in natural language (as e.g. OpenAI's o1 would).
Abstract from the paper:
Large language models (LLMs) are restricted to reason in the "language space", where they typically express the reasoning process with a chain-of-thought (CoT) to solve a complex reasoning problem. However, we argue that language space may not always be optimal for reasoning. For example, most word tokens are primarily for textual coherence and not essential for reasoning, while some critical tokens require complex planning and pose huge challenges to LLMs. To explore the potential of LLM reasoning in an unrestricted latent space instead of using natural language, we introduce a new paradigm Coconut (Chain of Continuous Thought). We utilize the last hidden state of the LLM as a representation of the reasoning state (termed "continuous thought"). Rather than decoding this into a word token, we feed it back to the LLM as the subsequent input embedding directly in the continuous space. Experiments show that Coconut can effectively augment the LLM on several reasoning tasks. This novel latent reasoning paradigm leads to emergent advanced reasoning patterns: the continuous thought can encode multiple alternative next reasoning steps, allowing the model to perform a breadth-first search (BFS) to solve the problem, rather than prematurely committing to a single deterministic path like CoT. Coconut outperforms CoT in certain logical reasoning tasks that require substantial backtracking during planning, with fewer thinking tokens during inference. These findings demonstrate the promise of latent reasoning and offer valuable insights for future research.
In January of 2026, this market will resolve YES if the state-of-the-art (SotA) reasoning model uses some latent space representation of its cognitive state to reason across multiple iterations before giving its final answer.
It doesn't count if the model merely manipulates latent space within a single forward pass (since all LLMs already do this). Loosely speaking, the model has to use its weights to get a latent vector, then reuse those same weights to process that latent at least once without generating any natural language tokens in between. If it uses some mix of latents and natural language in its reasoning, this still counts as using latent space.
I will primarily be looking at reasoning-centric evaluations such as FrontierMath and GPQA to determine which model is the SotA. Ultimately, the resolution will be based on my best judgement. I will not trade in this market.
Gemini tech lead making some pretty bullish comments about this here:
See timestamp 44min. He "doesn't want to taboo it" and likes the capabilities it can yield, and handwaves that we need interpretability but says future capabilities will probably make that easier